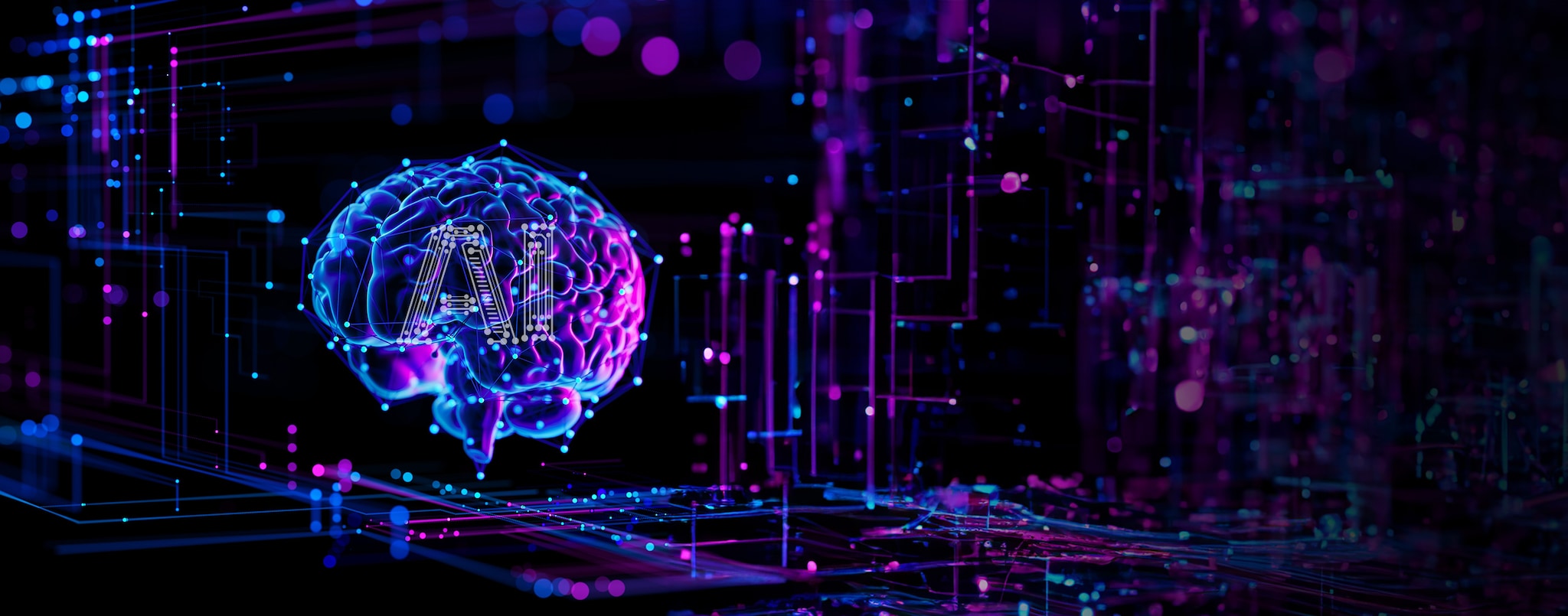
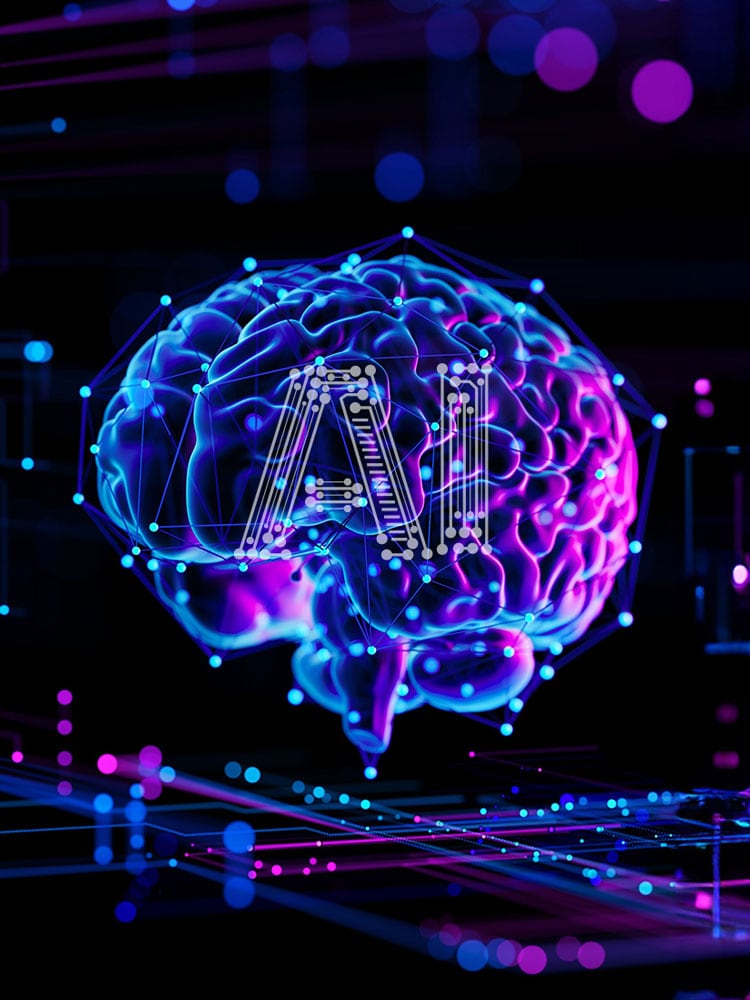
Cutting AI’s Power Consumption Down to 1/100 with Neuromorphic Devices Inspired by the Human Brain
Neuromorphic computing refers to a type of computing system designed to mimic the neural networks of the human brain using electronic components and circuits. Neuromorphic computing is a next-generation technology that can dramatically reduce power consumption, a challenging feat for traditional computing systems. By leveraging spintronics—a core technology of TDK—the company has developed the spin-memristor, an element that plays a foundational role in neuromorphic devices. TDK is working towards actualizing neuromorphic devices capable of reducing the power consumption of today’s AI systems to less than 1/100 of current levels.
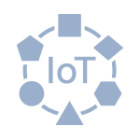
Explosive adoption of generative AI is threatening power supply globally
Since the 2022 public release of ChatGPT, U.S.-based OpenAI’s conversational AI, companies worldwide across a wide range of industries have been embracing generative AI, resulting in its explosive utilization. This enormous surge in demand for generative AI has fueled the growth of related industries like data centers and semiconductors. The rise of generative AI, in addition to the currently dominant cloud-based processing model, is expected to drive the growth of edge AI—where AI processing occurs under restricted power availability, such as in cars, on mobile phones, and near sensors—further expanding the market. Cloud-based AI requires power not only for AI processing but for data transmission as well. With edge AI, sensor signals can be converted locally into usable information—implying massive power savings when considering AI on the scale of becoming a part of our social infrastructure.
As generative AI continues to spread at a remarkable pace, a growing concern is the increasing power consumption associated with the rising demand. If AI usage continues to grow at its current rate, global energy consumption is predicted to skyrocket: for example, a report published by the International Energy Agency (IEA) indicates that, in 2022, data centers, AI, and related technologies consumed around 460 TWh (terawatt-hours) globally. By 2026, this figure could more than double, reaching almost 1,000 TWh. This level of consumption is comparable to the total energy usage of Japan—the world’s third-largest economy by GDP—making this a pressing societal issue. Consequently, pressure is intensifying within society to reduce the power consumed by AI usage.
Global electricity demand from data centers, AI, etc., as forecasted by the IEA
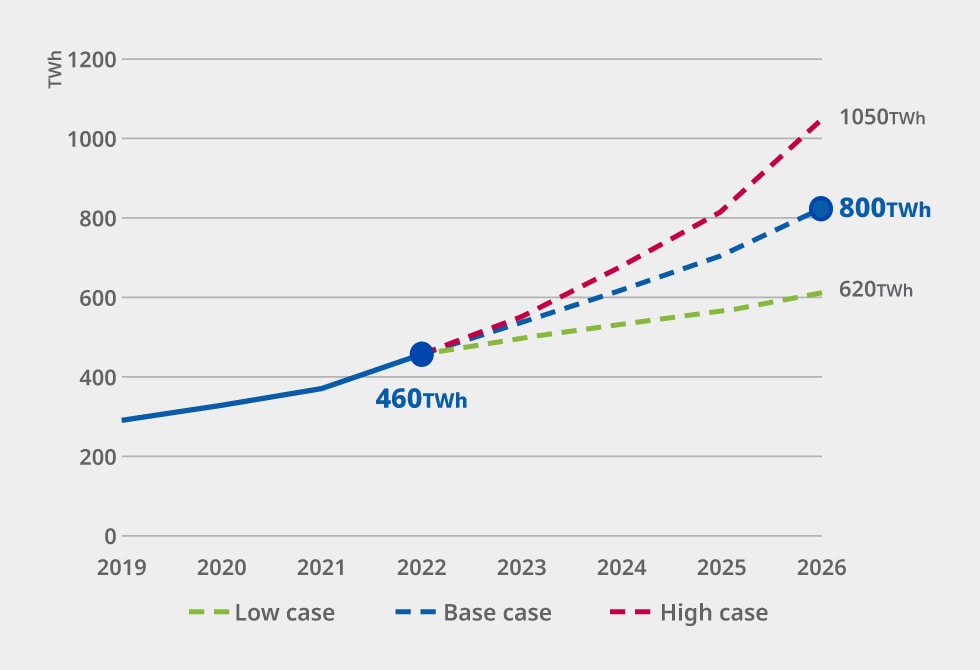
Neuromorphic devices can fundamentally reshape computing but face technical challenges
Because semiconductor performance improvements are approaching their physical limits*1, atop other adverse factors, achieving lower power consumption in AI processing calls for groundbreaking technologies—the kinds that fundamentally overhaul the architecture of computers. Today’s computers are predominantly based on the von Neumann architecture*2, which stores data and programs in memory prior to execution. But it is encumbered by an inherent drawback: since the memory and processing units are separate, moving data between them consumes a significant amount of power. Enter neuromorphic computing: a next-generation alternative to von Neumann that is emerging as a promising solution. This approach mimics the electrical behavior of neural networks in the human brain using electronic (integrated) circuits.
The human brain can function on roughly 20 watts of power and is said to be capable of making far more complex decisions using only about 1/10,000 of the energy consumed by today’s digital AI processing. Neuromorphic computing, which integrates memory elements directly into the processing units like the human brain, eliminates the need for data transfer. This allows for significantly faster data processing and reduced power consumption compared to the von Neumann model. This is why neuromorphic computing is garnering high expectations for AI applications across a variety of devices and systems.
Neuromorphic devices, which electrically mimic neurons (nerve cells) and synapses (the connections between neurons) in the human brain, have been under development by companies and research institutions worldwide. However, they have faced formidable technical challenges, particularly with memristors*3, which are used as the building blocks of a neuromorphic device. Conventional memristors have been notoriously difficult to work with due to their complex response behavior and issues like stored resistance values drifting over time. These problems have persisted as major roadblocks to actualizing neuromorphic technology.
Spin-memristor was developed through the application of spintronics technologies
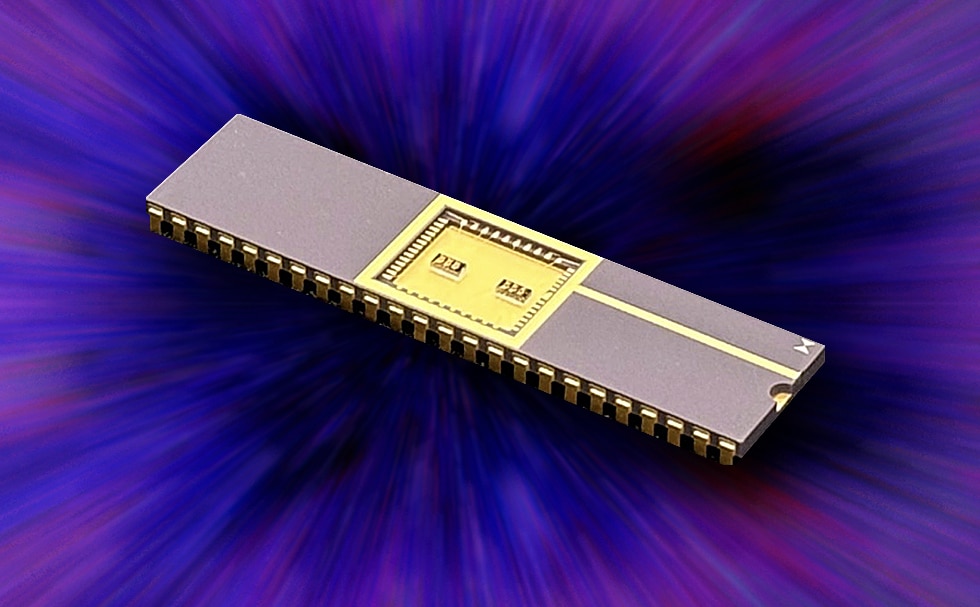
In response to these challenges, TDK developed the spin-memristor, an electronic element that leverages magnetics and spintronics. By expanding on spintronics*4—one of TDK’s core technologies behind its flagship products like magnetic heads for hard disk drives and TMR sensors—the company successfully overcame the issues plaguing conventional memristors. This new memristor offers straightforward, easy-to-use response characteristics, maintains stable resistance values over time, and enables high-speed response with low power consumption.
(Photo: Spin-memristor developed by TDK in a ceramic package)
In collaboration with the French Alternative Energies and Atomic Energy Commission (CEA), the spin-memristor has been successfully demonstrated to function as a basic element of neuromorphic devices.
Power consumption compared to a GPU
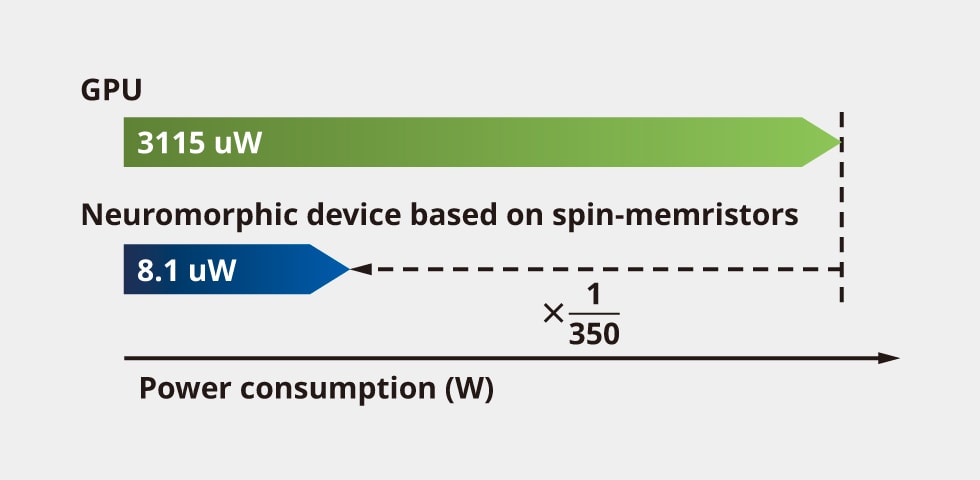
A device based on the von Neumann architecture is typically fabricated using mainstream CMOS semiconductor technology, where power efficiency is improved primarily through miniaturization and often only incrementally. Neuromorphic devices based on spin-memristors can achieve far superior power savings.
Looking ahead, TDK plans to work with Tohoku University’s International Center for Integrated Electronics to bring to practical use neuromorphic devices that can reduce power consumption down to less than 1/100. Tomoyuki Sasaki, Section Head and Senior Manager of the Spintronics R&D Section at TDK’s Technology and IP Headquarters, along with Tatsuo Shibata, Leader and Manager of Neuromorphic Device Team, shared their thoughts on future prospects. “The spin-memristor project is now transitioning to a development stage aimed at practical application. This necessitates the integration of the semiconductor and spintronics fabrication processes, technologies we will be developing in collaboration with Tohoku University. Our goal is to create new AI devices that not only consume much less power but also learn in real time and adapt to diverse environments and users.”
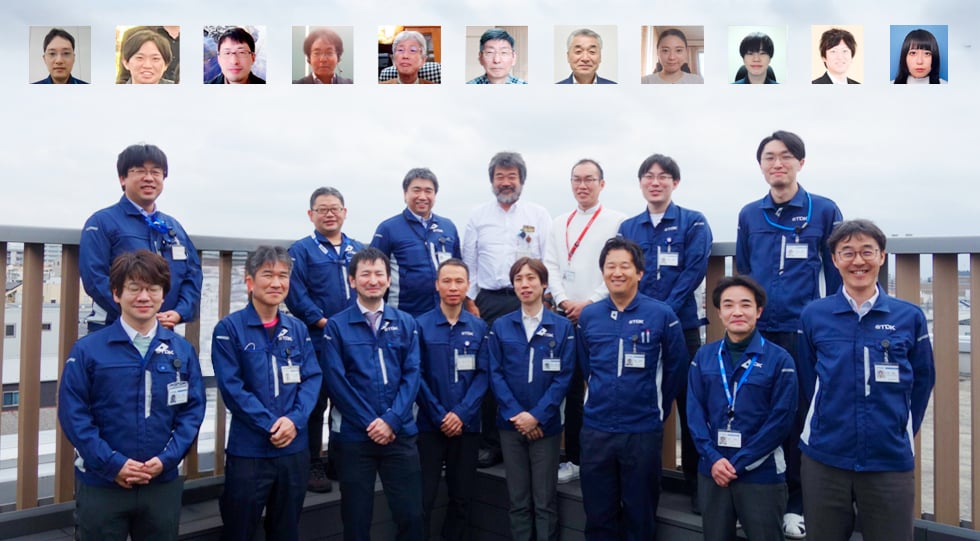
TDK is helping to build a more convenient and comfortable digital transformation (DX) society by driving the development of neuromorphic devices and reducing the power consumed by AI’s continued growth.
Demonstration circuit for voice separation featuring spin-memristors
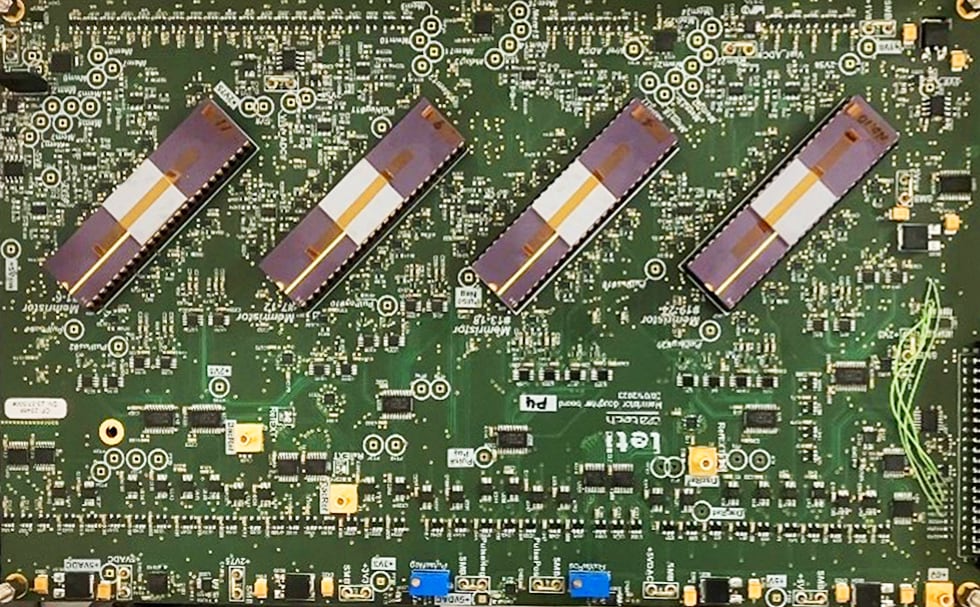
An AI circuit board equipped with spin-memristors encased in four ceramic packages. This demonstrated that spin-memristors can function as basic elements of a neuromorphic device.
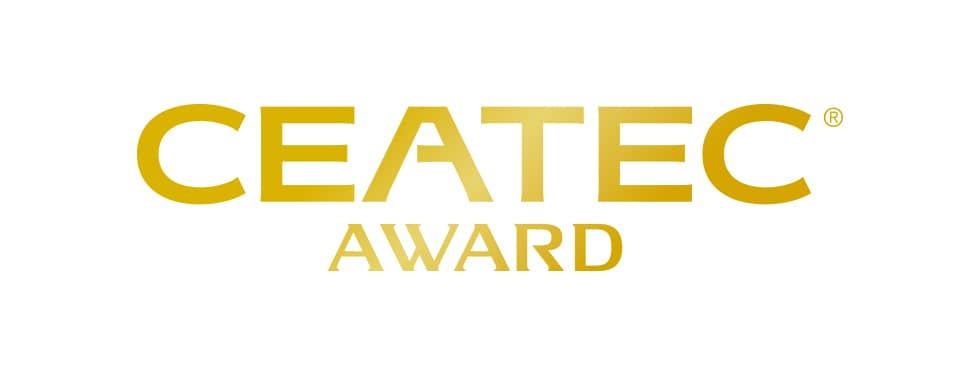
Terminology
- There is a growing belief in recent years that Moore’s Law, which predicts that the number of transistors in a semiconductor integrated circuit doubles every 18 (or 24) months, is reaching its physical limits.
- Von Neumann architecture: One of the fundamental operating models of a computer, where programs and data are stored in memory and executed sequentially.
- Memristor: A passive electronic component whose resistance changes based on the current that flows through it, allowing it to store the resistance value.
- Spintronics: A technology that utilizes both the charge and spin of electrons or the elements of spin.
